In this GLASE article, we talk about AI, its potential for controlled environment agriculture, and principles for adopting AI to horticulture.
The use of artificial intelligence in the production of controlled environment crops has the potential to grow crops more quickly and efficiently.
Most people are familiar with the term artificial intelligence or AI. Ken Tran, founder of Koidra LLC, said artificial intelligence is a very loosely defined term.
“AI very broadly means anything that a computer can do to perform a task,” said Tran. “Classical AI can be a program that doesn’t have the capability to learn and improve all the time. For example, a program can be written for a computer to play chess. A computer can play chess by itself, but it follows a specific logic imposed by the programmer. This type of AI doesn’t improve over time with data.”
A second type of AI can have accountability to learn and improve over time with additional data.
“This type of AI is very promising because it can continuously improve,” Tran said. “With this type of AI a computer can autonomously learn how to use the data.”
Tran said both types of AI are useful and will enhance each other.
“The second type is considered the second stage of AI,” he said. “The learnable AI is the next phase of the expert-system type of AI. Both types of AI could have major applications to controlled environment agriculture.”
Greenhouse challenge incorporates AI
Tran was the principal investigator and leader of a team of AI and horticulture experts that won the first International Autonomous Greenhouse Challenge organized by Wageningen University and Research in the Netherlands. Tran was the principal research engineer for the Project Sonoma team. At the time Tran participated in the challenge he was an employee of Microsoft Research where much of his focus was on machine learning.
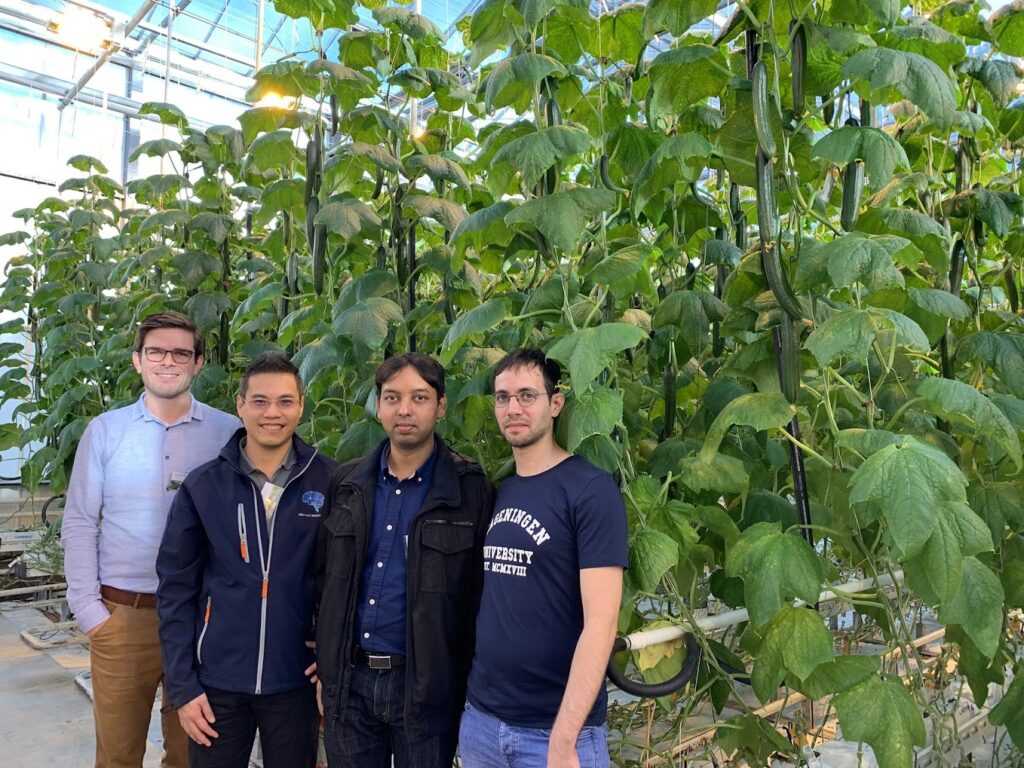
The greenhouse challenge ran from May through December 2018, with five teams growing a cucumber crop in their own greenhouse compartment at the university. The purpose of the challenge was to combine AI with greenhouse data to maximize crop production while minimizing greenhouse inputs.
“The success of the Sonoma team in the competition came from our collaboration with horticulture researchers,” Tran said. “Without their participation and the domain knowledge they provided, we wouldn’t have achieved this success.”
The team worked with researchers including Dr. Xiuming Hao at Agriculture and Agrifood Canada, Shalin Khosla at Ontario Ministry of Agriculture, Food and Rural Affairs Agriculture and Dr. Chieri Kobota at Ohio State University.
“Before growing cucumbers for the competition, we had not grown cucumbers, but we were still able to win,” Tran said. “During the competition, our team outperformed a team of expert Dutch growers who had previously grown cucumbers. Our team was able to produce more than 55 kilograms of cucumbers per square meter. Also, the net profit on the cucumbers was 17 percent higher than the Dutch growers.”
Potential benefits for CEA
While Tran was employed at Microsoft he worked on reinforcement learning. He explained reinforcement learning, in a simplistic definition, is a data-driven method used in control applications. It learns to find the best actions based on reward or punishment data.
“We wanted to find a good application to motivate our reinforcement learning research,” he said. “In 2017, I was looking for a pure application, meaning an application that would have a great impact and would also be doable for reinforcement learning. I began with indoor vertical farms which are a good application because it is a well-controlled environment with little influence from the outside. It is easy to get started because the test environment can be as simple as a small growth chamber.
“Some types of applications that would fit include using reinforcement learning to solve a control problem. We wanted to solve a problem in the real world, but applying reinforcement learning in the real world is really challenging. That is why I was looking for applications that are well controlled and can have different scales from a small growth chamber to a large grow room.”
Tran saw the potential that vertical farms had to help solve sustainable food production problems worldwide. He spoke with CEA researchers and experts around the world, including the United States, Japan and China. One of the institutions that Tran contacted was Wageningen University.
“When we were visited the university we learned about how they were going to organize this greenhouse challenge,” Tran said. “We discussed with them exploring collaborative opportunities. With this competition platform we could get our feet wet by actually doing something and not just talking about theory and the possibilities.”
Collecting more data from growers
Tran said much more data is needed from growers in order for computers to autonomously learn how to use the data.
“We are trying to understand how good growers produce a crop,” he said. “This isn’t just one grower, but multiple growers. We study the plant science and try to come up with a sound formula for how to grow a crop. That formula doesn’t evolve itself. It is a fixed formula. It is reacting to changing conditions that indicates under these conditions to try this.
“This is the first step in our research and it was very successful. It already performed better than many other growers would because we were able to aggregate the knowledge from multiple expert growers. We are trying to develop AI that can learn and improve over time with more data. We don’t want to stop at one system.”
Tran said controlling a vertical farm is easier than controlling a greenhouse.
“However, in both scenarios our current technology can already be used,” he said. “This technology will keep evolving for even further impact. The technology can be used in both applications by using what we already know about plant science, machine learning and AI in general. It’s not like having to wait for new technology or the research is not ready and we have to wait. We can already leverage the technology today and we have demonstrated that in multiple scenarios.”
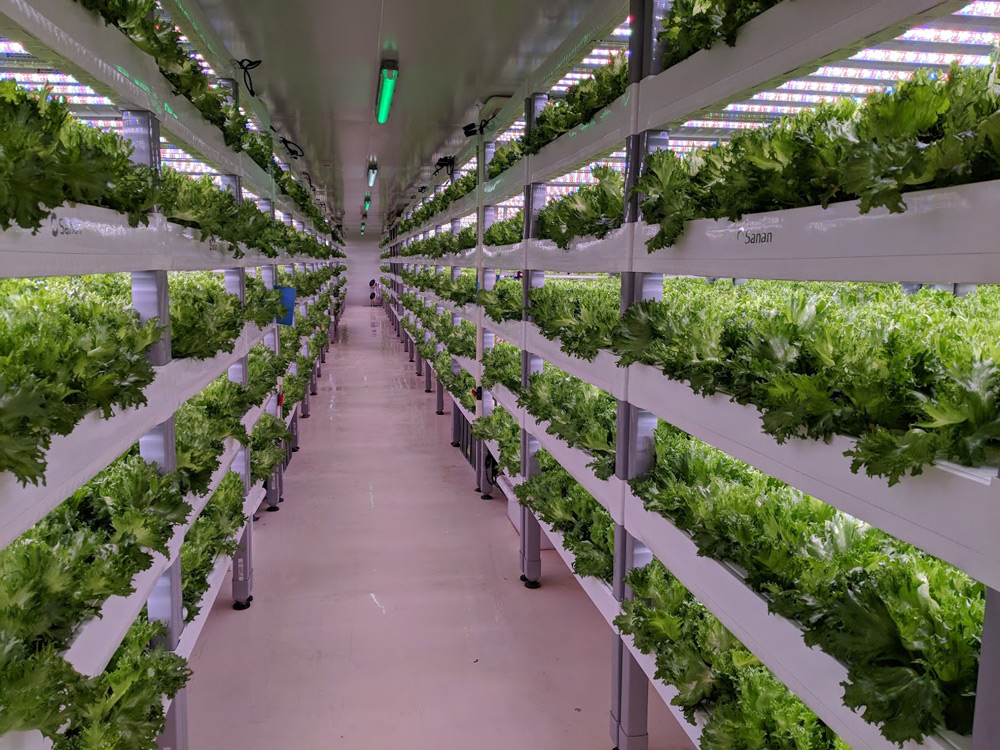
Tran is working on a commercial AI program that will be adaptable to a variety of crops.
“The process is going to be similar for developing a program for any CEA crop,” he said. “The data will include environmental data from inside and outside the greenhouse. This data will be generated automatically from multiple sensors installed inside the greenhouse, including light levels, temperature, relative humidity, nutrient levels, water quality and carbon dioxide levels.
“For every crop, we would need to talk with the growers to find out how they currently grow to set up a baseline. Crop data would be provided manually by the growers on a daily and/or weekly basis depending on the crop. The program will evolve from the baseline with more data coming in. We want the program to be safe for every crop.”
Principles for adopting AI to horticulture
Tran said in order for growers and the horticulture industry to adopt AI, safety-first principles must be followed. These include:
- The AI system must start growing the same way as what growers want with no risky deviations from what growers would do. This growing would continuously improve.
- Growers could easily switch between manual, recommendation and Copilot modes. The greenhouse operator is always in control and can choose to exit AI control mode at any time. In recommendation mode, the AI system would only send recommendations to the operator for review and the set points would still be inputted manually by the operator.
- The system must support easy and continuous monitoring.